Keras 신경망을 사용하여 학습한 callback 가중치를 model.load_weights(checkpoint_path) 를 사용하여 불러낸 후 별도의 unseen image 의 라벨 값을 예측(prediction) 해 보자.
예측하고자 하는 하나의 이미지 즉 여기서는 diffenbachia 잎사귀 사진을 사용하였다.
이를 읽어 들이기 위해서 OpenCV imread 명령을 사용하자.
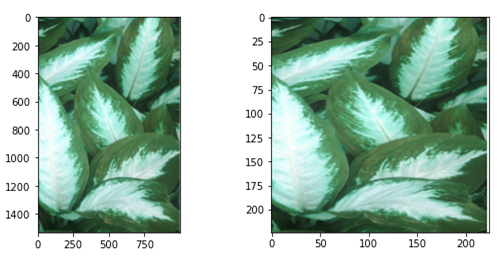
실제 이미지의 해상도는 고해상도이지만 classification 목적을 위해서 학습과정에서 사용했던 표준적인 (224,224) 로 resize 한다.
1 | import cv2 data_root = ("D:/⦁⦁⦁/ImageClassfication/DataSet") img_height = 224 img_width = 224 batch_size = 15 data_dir = str(data_root) #Load image by Opencv2 img = cv2.imread('diffenbachiaex.jpg') img = cv2.resize(img, (img_width , img_height )) |
resize 된 이미지를 tensor 또는 np.array 로 변환하고 batch = 1 에 해당하도록 tensor 의 shape을 하나 증가시켜 (1, 224, 224, 3) 로 만든다.
2 | # img_tensor = tf.convert_to_tensor(img, dtype=tf.uint8) img_tensor = np.array(img) unseen_image = tf.expand_dims(img_tensor , 0) print('unseen_image.shape : ', unseen_image.shape) |
data_root 폴더에 분류를 위한 데이터세트를 저장하고 keras 신경망을 사용하여 학습시키자. 학습된 가중치를 저장한 후 업로딩하여 재사용하려면 callback 정보 저장 경로를 설정하고 model.fit 명령에서 callback 파라메터를 사용하도록 한다.
학습 완료 후 callback 된 가중치를 업로딩하여 검증 데이터세트와 학습데이터세트를 re-evaluate 해보자. 검증 데이터는 70% 수준, 학습데이터는 당연히 100% 가 되어야 할 것이다.
3 | ⦁⦁⦁ model = create_model(num_classes) # Loads the weights model.load_weights(checkpoint_path) print('valid_label_batch :', valid_label_batch[0].numpy()) loss, acc = model.evaluate(valid_image_batch, valid_label_batch, verbose=2) print("Untrained model, accuracy: {:5.2f}%".format(100 * acc)) loss, acc = model.evaluate(train_image_batch, train_label_batch, verbose=2) print("Already trained model, accuracy: {:5.2f}%".format(100 * acc)) |
tensor 처리된 unseen_image를 사용하여 model.predict 명령을 실행하면 (1, num_classes) shape 을 가지는 predictions 가 얻어지며 shape=(1,6) 에서 axis=0 을 기준으로 argmax 값을 얻어 calss_names 에 물어보면 2번째 ‘diffenbachia’ 라는 답을 예측해준다.
4 | predictions = model.predict(unseen_image) print('predictions.shape : ', predictions.shape) print(predictions) predicted_class_index = np.argmax(predictions[0]) predicted_class = class_names[predicted_class_index] print('Predicted class:', predicted_class) |
아래의 print(predictions) 출력 결과를 살펴보면 두 번째 diffenbachia 확률이 0.9956 임을 알 수 있다.
첨부된 diffenbachia 이미지와 코드를 다운받아 실행해 보자.
'인공지능 응용 공학' 카테고리의 다른 글
인공지능응용 공학∙ OpenCV 비전코딩 목차 pdf 파일 (0) | 2023.06.14 |
---|---|
No validation prediction of unseen image using callback weights (0) | 2023.06.14 |
OpenCV 명령을 응용한 하네스 커넥터 확대 이미지의 의 윤곽선 추출 (0) | 2023.06.07 |
Keras 학습가중치 callback 업로딩에 의한 이미지 분류 (0) | 2023.06.07 |
tf.keras.preprocessing.image.ImageDataGenerator 에서 tf.keras.utils.image _dataset_from_directory 로 변경하여 이미지 분류 (0) | 2023.06.07 |